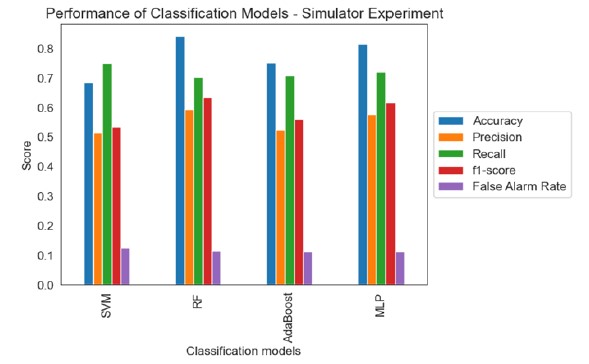
Road safety is a subject of significant concern and substantially affects individuals across the globe. Thus, real-time, and post-trip interventions have gained significant importance in the past few years. The European Union’s Horizon 2020 project i-DREAMS has also directed its attention towards this aspect. In particular, i-DREAMS aimed to define, develop, test, and validate a ‘Safety Tolerance Zone (STZ)’ in order to prevent drivers from risky driving behaviors using interventions both in real-time and post-trip. This study aimed to analyze different classification techniques and examine their ability to identify dangerous driving behavior based on a dual-approach study. The analysis was based on the investigation of important risk factors such as average speed, harsh acceleration, harsh braking, headway, overtaking, distraction (i.e., mobile phone use), and fatigue. In order to achieve the objective of this study, significant data were collected through a driving simulator as well as a naturalistic driving experiment. Based on the data collected for each of the two approaches, several classification models were developed, analyzed, and compared, according to their performance. To that end, four classification algorithms, namely Support Vector Machines (SVMs), Random Forest (RFs), AdaBoost, and Multilayer Perceptron (MLP) Neural Networks were implemented. The proposed methods were compared based on different evaluation metrics and it emerged that RFs and MLPs outperformed the rest of the classifiers with 84% and 82% overall accuracy, respectively, while the maximum average speed of the vehicle was found to be the most crucial predictor for identifying the driving time at each safety level. Risky and aggressive driving behavior is a worldwide critical social and public health concern. The findings of this research could provide essential guidance for decision-makers to initiate concrete steps for engineering applications in road safety management.
ID | pc537 |
Presentation | |
Tags |