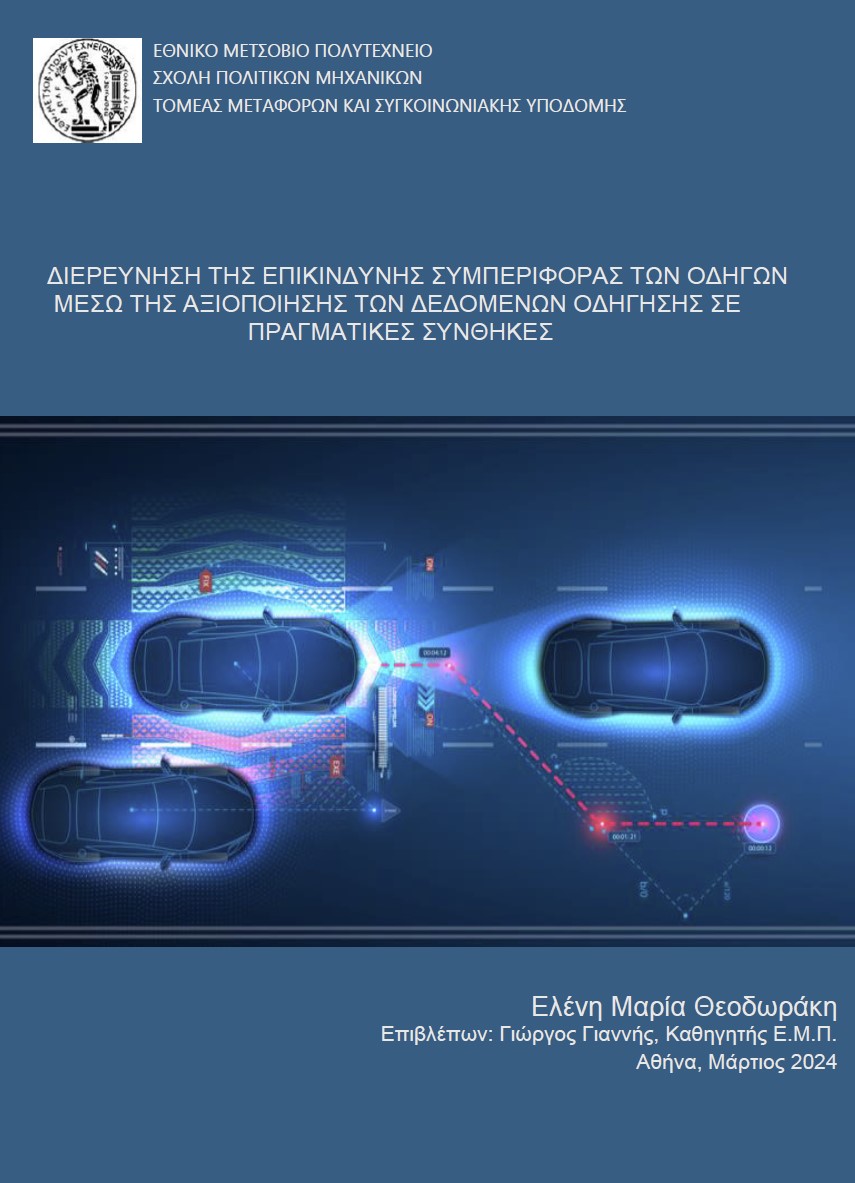
The present research aimed to develop classification models for the identification of dangerous driver behaviour at three safety levels. For this purpose, useful data related to driver behaviour were collected through a naturalistic driving experiment in real-life conditions in Belgium and England. Based on the processing and analysis of the data, three levels of risk were defined. In the first part of the analyses, three combined machine and deep learning algorithms were developed to classify driver behaviour into one of the three safety levels, with the ‘Deep Neural Network (DNN)-Reflective Forest (RF) model performing the best for both countries. As part of the investigation of the influence of driving factors on the identification of dangerous driving, the most important factors were found to be total travel distance, speed, occurrence or not of sudden acceleration and occurrence or not of sudden braking. The second part of the analysis examined the influence of driving characteristics on the prediction of the combined models by developing the Lime algorithm (Local Interpretable Model-agnostic explanations). The results showed that the most significant variables were the occurrence or non-occurrence of sudden acceleration and the occurrence or non-occurrence of sudden braking in Belgium and the total travel distance and the occurrence or non-occurrence of sudden acceleration in England.
ID | ad161 |
Presentation | |
Full Text | |
Tags |