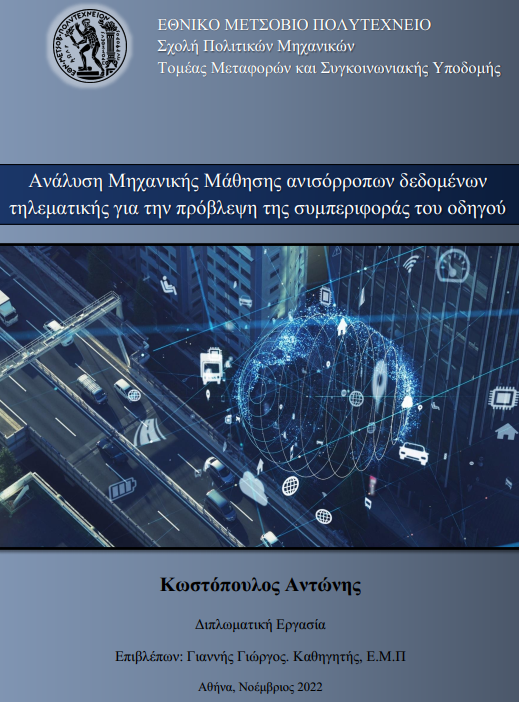
The objective of this Diploma Thesis is the exploitation of imbalanced learning for the task of classifying and predicting driving behaviour and harsh events, using naturalistic driving data. Data was collected through the telematics company OSeven, in order to classify and predict driving behaviour in terms of harsh accelerations and brakings occurences. More precisely, this thesis
intends to determine the most crucial predictors for the occurrence of harsh events, through a feature selection process and to identify two safety levels for harsh accelerations and brakings using Machine Learning techniques. K-means clustering revealed that users with more than 48 harsh accelerations and more than 45 harsh brakings per 100 km of driving were deemed the most dangerous. The imbalanced classification results showcased that the total driving distance was the more impactful variable to harsh events occurence, whilst the best techniques for this particular imbalanced learning process, were achieved by Gradient Boosting and Multilayered Perceptrons algorithms.
intends to determine the most crucial predictors for the occurrence of harsh events, through a feature selection process and to identify two safety levels for harsh accelerations and brakings using Machine Learning techniques. K-means clustering revealed that users with more than 48 harsh accelerations and more than 45 harsh brakings per 100 km of driving were deemed the most dangerous. The imbalanced classification results showcased that the total driving distance was the more impactful variable to harsh events occurence, whilst the best techniques for this particular imbalanced learning process, were achieved by Gradient Boosting and Multilayered Perceptrons algorithms.
ID | ad132 |
Presentation | |
Full Text | |
Tags |