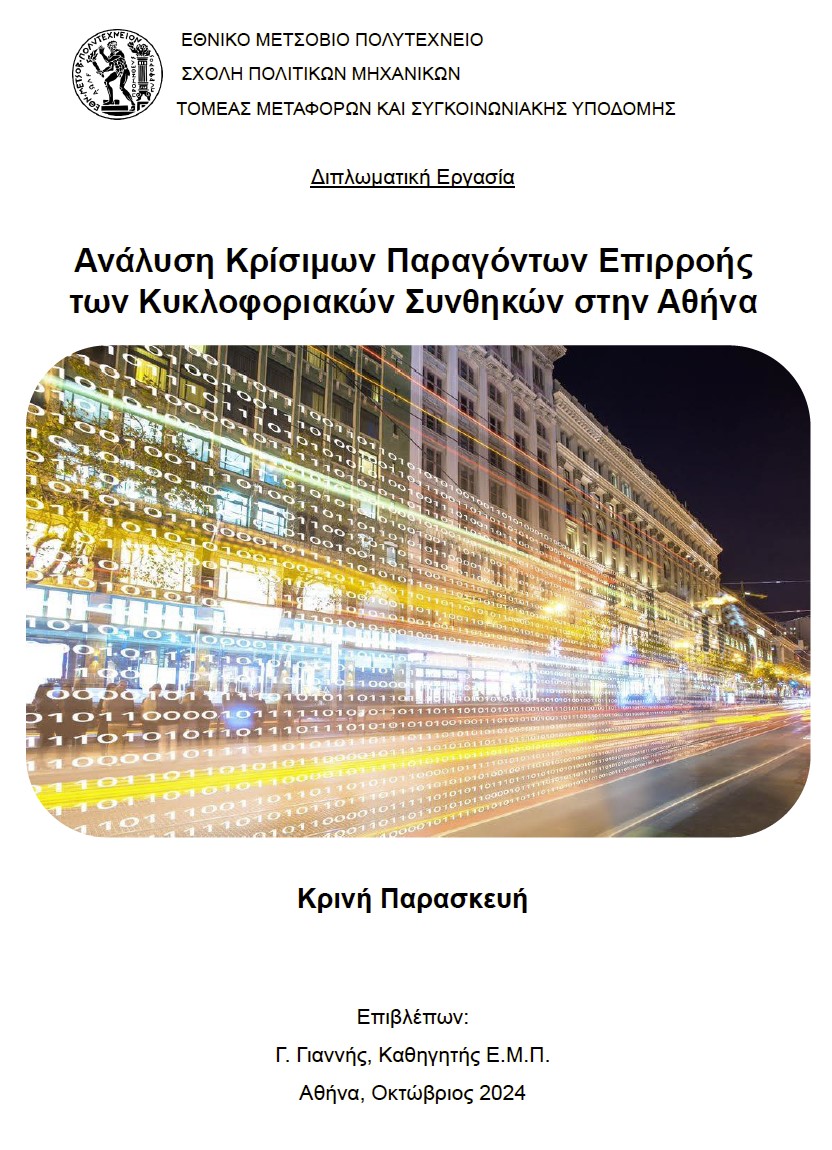
The aim of this thesis is to analyse the critical factors influencing traffic conditions in Athens. More specifically, it aims to investigate the multifaceted nature of traffic congestion and highlight the parameters affecting it, such as weather conditions, time period (hour, day), and geometric characteristics of (road axes). For this reason, traffic data was collated from selected road segments in Athens, including traffic speed through Google Maps for 62 (road axes) and the hourly traffic volume at 26 points via the Centre for Traffic Management, covering the period from January to July 2022. Additionally, data on weather conditions were gathered, and the final database was completed with geometric road features. In the first phase, the relationship between traffic speed – traffic volume was examined, along with the evolution of traffic under different weather and time conditions. Subsequently, a CatBoost Machine Learning algorithm was developed to discover the degree of influence of each variable under investigation on traffic, specifically concerning hourly traffic volume and traffic speed. Then, five Cluster Analyses were conducted which aimed to identify clusters based on observed traffic conditions under various environmental and time factors, using the K-means method. The application of the models revealed that traffic metrics are significantly related to the time and type of day (weekday/weekend), with peak traffic volume occurring on weekdays during morning rush hours. Moreover, an increase in temperature is associated with a decrease in traffic volume in the morning and an increase in the evening, while the presence of rain increases low volume of traffic and decreases high traffic speeds.
ID | ad166 |
Presentation | |
Full Text | |
Tags |