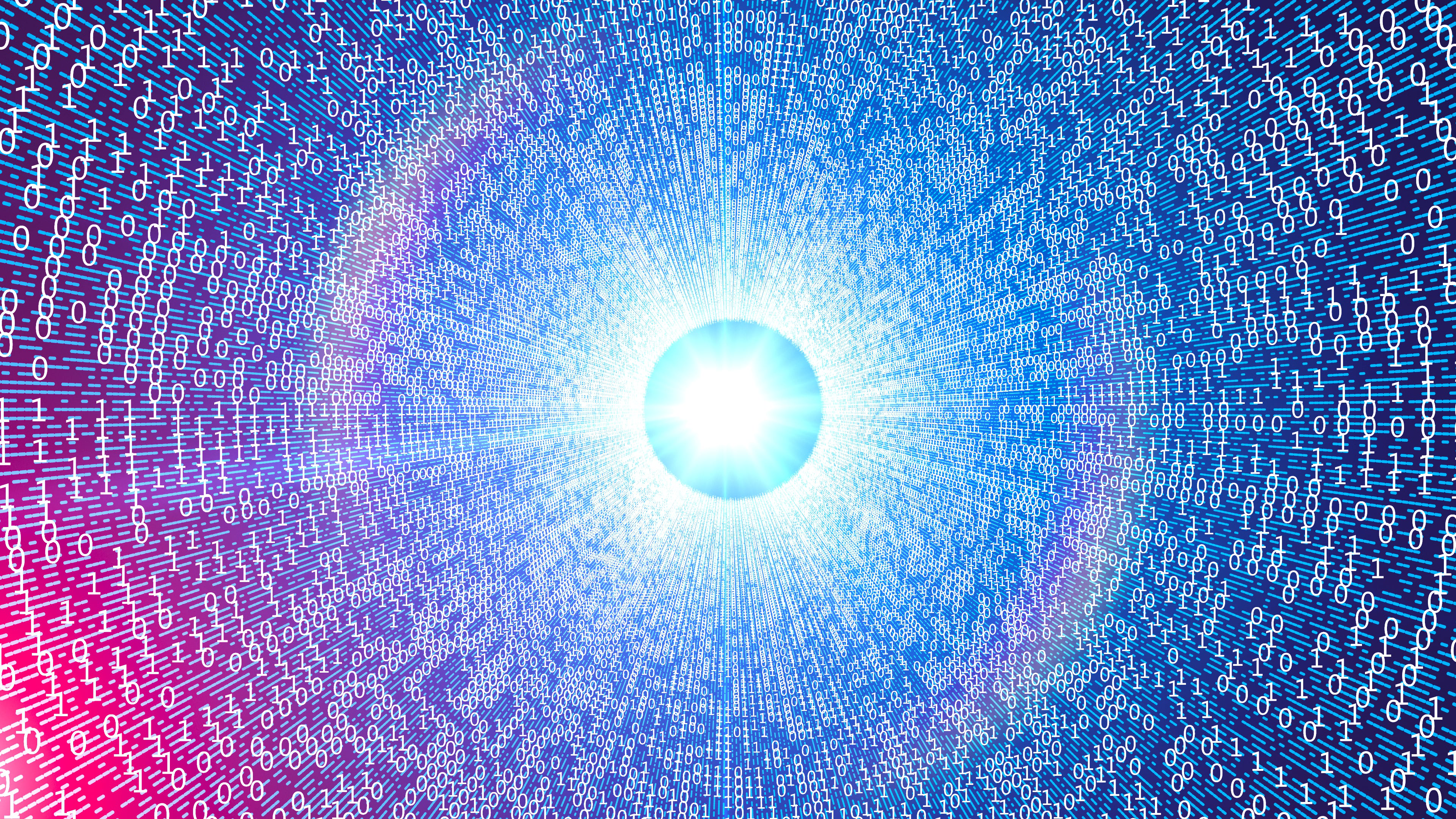
• To create an AI framework to process, harmonise, analyse and model an array of different available datasets and provide outputs in the form of risk mapping and network-level evaluations
• To develop new AI algorithms for road attribute collection, along with methodologies to assess and quantify their accuracies, suitable for network applications and including hybrid, e.g., manually collected data
• To use the AI-augmented dataset creation effort for a suitable working methodology for the generation of hybrid road attribute data and enhanced proactive risk mapping
• To develop new AI algorithms for road attribute collection, along with methodologies to assess and quantify their accuracies, suitable for network applications and including hybrid, e.g., manually collected data
• To use the AI-augmented dataset creation effort for a suitable working methodology for the generation of hybrid road attribute data and enhanced proactive risk mapping
• A functional framework with the use of AI to exploit road risk information in a meaningful manner, transferable between networks
• Assessment and quantification of the influence of each examined factor on the output of AI algorithms for safety management
• A case study with actionable results, compatible with the mission and activities of iRAP – namely, a proactive risk mapping and evaluation of a network and comparison with existing risk-mapping results, and quantification of transferability to a second network
ID | at21 |
Tags |