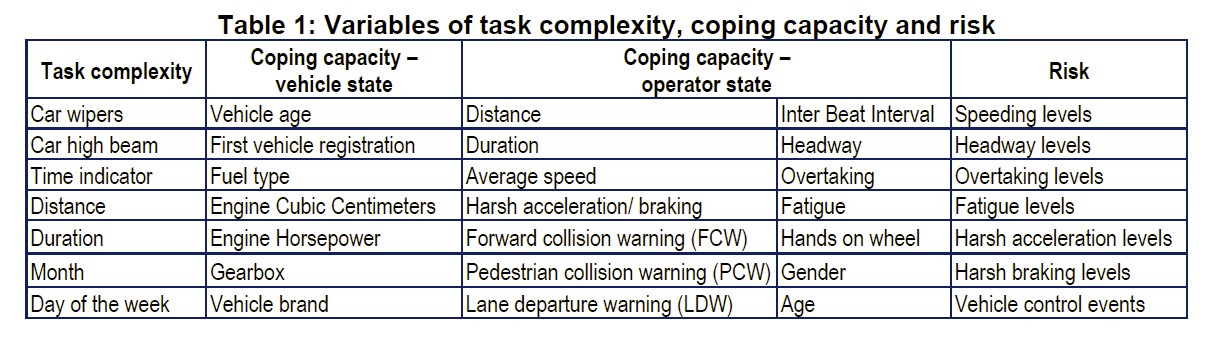
Human behaviour plays a pivotal role in road safety. Factors such as speeding, distraction or aggressive driving can elevate crash risk. Moreover, the complexity of driving task, such as weather conditions, traffic density or road infrastructure and vehicle conditions have also a significant impact on risk. The aim of this paper was to quantify the impact of driver, vehicle and environment on crash risk using big data. Towards that end, a naturalistic driving experiment was taken place and data from 135 drivers aged 20-65 were collected and analysed. Towards that end, Generalized Linear Models (GLMs) were developed and explanatory variables of risk with the most reliable indicators, such as time headway, distance travelled, speed, time of the day or weather conditions were assessed. Additionally, Structural Equation Models (SEMs) were used to explore how the model variables were inter-related, allowing for both direct and indirect relationships to be modelled. The analyses revealed that drivers, when faced with difficult conditions, tend to regulate well their capacity to apprehend potential difficulties, while driving. It was also found that complex environment conditions led to an increased crash risk due to several reasons. The relationship among environment conditions, driver behaviour and vehicle situation with risk, may depend on the specific context and type of task or activity involved. Authorities may use data systems at population level to plan mobility and safety interventions, set
ID | pc551 |
Presentation | |
Full Text | |
Tags |