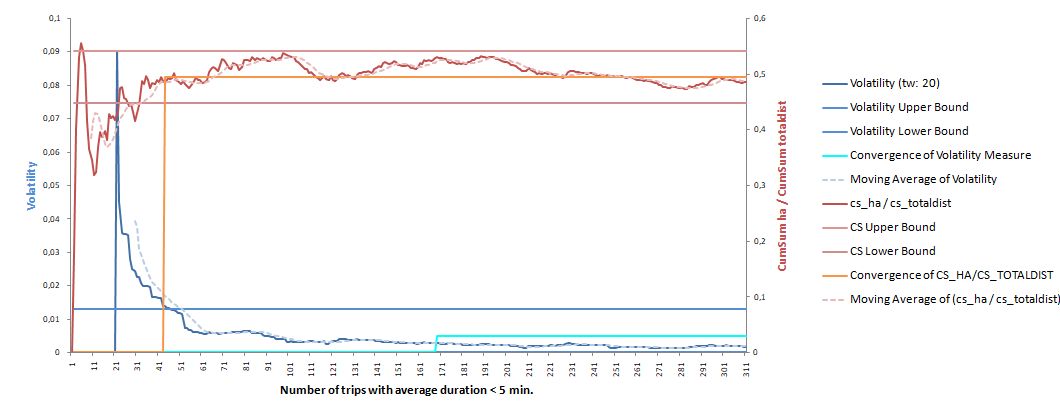
The aim of this study is to provide a methodological framework for estimating the amount of driving data that should be collected for each driver in order to acquire a clear picture regarding driving behavior. This amount is defined as the total driving duration and/or the number of trips that need to be recorded for each driver in order to draw a solid conclusion regarding where the rate of driving behavioral characteristics (e.g., per kilometer or per minute) has converged to a fixed point.
Several studies have taken advantage of new technologies such as In-Vehicle Data Recorders and smartphones for the evaluation of driving behavior (1-5). However, the exact amount of driving data that need to be collected and evaluated to assess driving behavior with sufficient precision has not yet been determined. Both small and large data samples are likely to lead to questionable results by acquiring a sample either biased or computationally expensive to analyze, and thus, it is important to investigate the amount of driving data that should be recorded by each participant in the experiment.
In this study, the driving metrics used to identify driving behavior stabilization are the number of harsh acceleration and braking events, the time of mobile phone usage and the time driving above the speed limit (speeding), which are the main human factors used in literature as well (6-9). Through the exploitation of cumulative sums, moving averages and Shewhart control charts, the driver’s aggression and volatility is estimated, and consequently, the time point at which driving behavior converges is determined. The analysis indicated that for a certain driving characteristic, convergence depends largely on the aggressiveness and stability of the overall driver’s behavior as well as the average duration of the trips being studied. The results of the analysis performed could be exploited both by the private and public sector, providing multiple social and economic benefits.
ID | pc325 |
Presentation | |
Full Text | |
Tags | big data, driver behaviour, machine learning, naturalistic driving, statistical modelling, telematics |