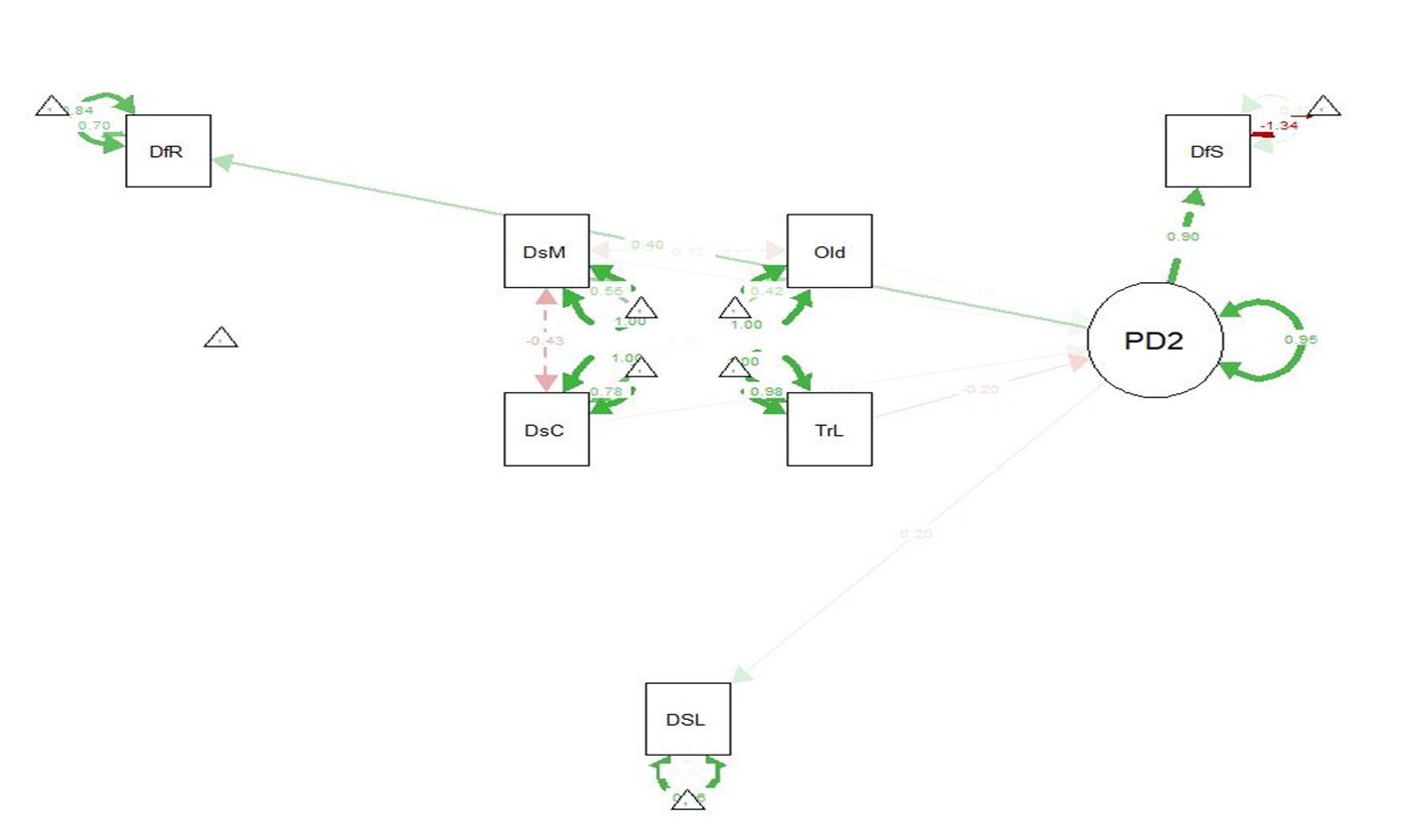
Extended Abstract
Problem statement
While road accidents constitute a major social problem in modern societies, accounting for more than 1.2 million fatalities worldwide and 25.500 in the European Union in 2015 (WHO, 2017), unexpected events are part of everyday driving experience and consist a key parameter of road accidents as they lead to motor slowing and cognitive distraction (Wessel and Aron, 2016). When experiencing an unexpected event, the driver temporarily switches from normal driving to compensatory driving actions (Schaap et al., 2008). While different varieties of unexpected events have been studied largely independently, many different mechanisms are thought to explain their effects on driving perfomance.
In order to investigate unexpected events, a very useful tool is driving simulators mainly due to the fact that they allow the examination of a range of driving performance measures in a controlled, relatively realistic and safe driving environment. Within this framework, a large range of test conditions can be implemented in the simulator with relative ease, and these conditions can include hazardous or risky driving situations that would be too difficult or dangerous to generate under real driving conditions such as unexpected incidents and distraction sources (Regan et. al., 2008).
In addition, driving performance is a multidimensional phenomenon which means that no single driving performance parameter can capture all aspects of the overall driving performance. However, in the majority of studies where the research question is the investigation of driving performance, individual driving parameters are considered to represent performance. Instead, an unobserved (latent) new variable can be developed based on the collected individual parameters and represent with a statistical significance the overall driving performance.
Research objectives
The present research relies on two objectives. The first is to investigate whether latent model analysis through a structural equation model can be implemented on driving simulator data in order to define an unobserved variable which reflects the difference of driving performance before/after an unexpected event. Subsequently, the second objective is to investigate and quantify the effect of several risk factors including distraction sources, driver characteristics, road and traffic environment on the difference of overall driving performance and not in independent parameters.
Methodological approach
Within this framework of the present research, a driving simulator experiment was carried out, in which 95 participants were asked to drive under different types of distraction (no distraction, conversation with passenger, cell phone use) in different road (urban/rural) and traffic conditions (high/low). Each participant aimed to complete 12 driving trials. Participants were also asked to fill in two questionnaires regarding their driving behaviour, as well as self-assessment and memory tests. The above stages were designed based on specific parameters and criteria as well as design principles that were appropriate for the research assumptions and objectives of the research.
During each trial of the experiment, 2 unexpected events were scheduled to occur at fixed points along each drive (but not at the exact same point in all trials, to minimize learning effects). More specifically, events in rural area concerned the sudden appearance of an animal (deer or donkey) on the roadway and events in urban areas concerned a car getting out of a parking position, or the sudden appearance of an adult pedestrian or of a child chasing a ball on the roadway.
The experiment data storage was performed automatically at the end of each experiment. The simulator recorded data at intervals of 33 milliseconds (ms). In order to achieve the scope of the present research the database was transformed so that one line referred to each event for all participants. In addition, the average value of all driving performance measures was estimated for a time period of 15 seconds before and 15 seconds after the event and a new column was estimated referring to the difference of each measure. As a result, the final database consisted of 931 rows (as the total number of unexpected events) and 81 parameters. Then, within the framework of the statistical analysis, through the implementation of latent model analysis, in the first step the difference of driving performance is defined as a new, unobserved variable, based on specific driving simulator parameters while in the second step the effect of distraction, driver as well as road and traffic characteristics are estimated directly on this new driving performance variable (instead of being estimated on individual driving performance parameters).
Results
Model results indicate that in the measurement part of the Structural Equation Model, the difference of driving performance (the latent variable) is correlated with the difference of average speed the difference of the variability of lateral position and the difference of motor revolvation. Consequently, considering that the examined statistical analysis is statistically acceptable, the quantification of the effect of several risk factors on the difference of the overall driving performance after an unexpected event is achieved through the structural part of the model. More specifically, both examined distraction factors were found to negatively affect the latent variable indicating that while conversing with the passenger or talking on the cell phone during an unexpected event, driving performance is less affected after the event. On the other hand, older drivers were found to change more their driving performance due to an unexpected event. In conclusion, the findings of this study allow a new approach on the investigation of driving behaviour in driving simulator experiments and in general when focusing on unexpected events.
ID | pc338 |
Presentation | |
Full Text | |
Tags | driver behaviour, driving simulator, statistical modelling |