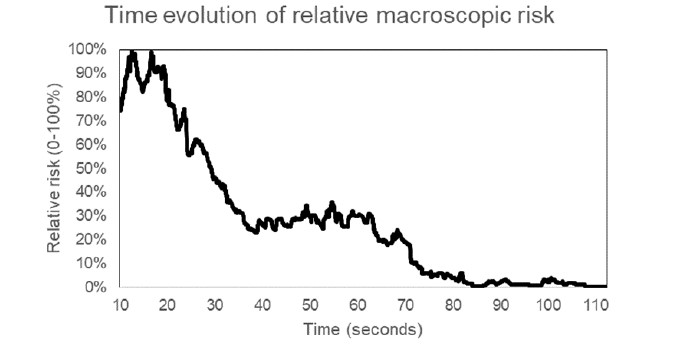
Accurate estimation of driving risk is essential for improving urban road safety and reducing crashes. 2 Despite advancements, a significant gap exists in the real-time estimation of microscopic driving risk 3 using advanced data technologies. This study presents an AI-based framework for assessing microscopic 4 driving risk using the pNEUMA dataset, which comprises drone-collected traffic data from Panepistimiou 5 Street, a five-lane urban arterial in Athens, Greece. Over five days, ten drones captured three hours of 6 traffic data daily. The study aims to predict the probability of the ego vehicle encountering risk-related 7 events such as speeding, lane changing, harsh braking, harsh acceleration, and short time-to-collision 8 scenarios. The proposed methodology employs Long Short-Term Memory (LSTM) neural networks, both 9 uni-directional and bi-directional, to analyze and predict driving risks based on the behavior of 10 surrounding traffic. LSTM models showed high precision in identifying risk-related events, 11 demonstrating the effectiveness of deep learning techniques in this context. Additionally, the results were 12 aggregated at the road section level, allowing real-time risk estimation across different arterial 13 subsections. This aggregation supports the development of real-time monitoring systems that provide 14 actionable feedback to drivers, thereby enhancing road safety. The findings highlight the potential of 15 integrating comprehensive data sources like drones to monitor and assess real-time driving behavior using 16 advanced AI techniques. This approach offers granular insights into both micro and macroscopic driving 17 risks. The study paves the way for future research into the application of similar methodologies in various 18 urban settings, ultimately aiming to develop comprehensive, real-time road safety systems.
ID | pc555 |
Presentation | |
Full Text | |
Tags |