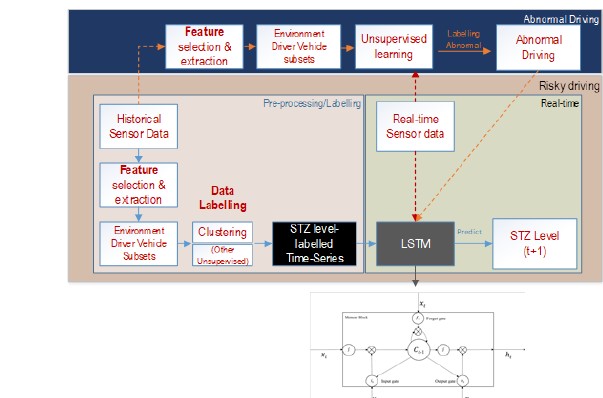
A wide range of variables affect road safety, including the driver’s state, environment, and traffic 2 conditions. The aim of the current study was twofold; a) the evaluation of the impact of various indicators 3 on predicting Safety Tolerance Zone (STZ) and b) the development of a deep learning model for 4 identifying dangerous driving. In order to achieve these objectives, a naturalistic driving experiment was 5 implemented and data from a representative sample of 50 Belgian car drivers were collected and 6 analysed. The impact of the features on the estimation of STZ was performed based on the XGBoost 7 algorithm. Key features such as headway, forward collision warning indicator, and distance traveled were 8 found to significantly affect the prediction of STZ levels. Subsequently, a Long Short-Term Memory 9 (LSTM) model was developed for real time data prediction, leveraging its strength in handling sequential 10 data and temporal dependencies. The results demonstrated that the LSTM model achieved a 71% 11 accuracy rate in identifying dangerous driving behaviors, underscoring the potential of this approach for 12 enhancing road safety. The analysis highlighted the critical importance of the identified features, 13 suggesting that the combination of XGBoost for feature selection and LSTM for real-time prediction 14 provides a robust framework for real-time intervention and support systems. This integrated method 15 offers significant promise for reducing road accidents and improving overall traffic safety by enabling 16 timely and accurate identification of risky driving behaviors.
ID | pc556 |
Presentation | |
Full Text | |
Tags |