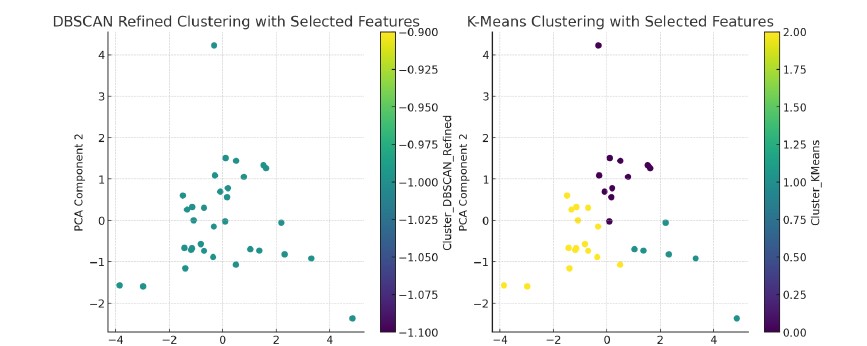
This research explores the relationship between unsafe driving events and crash occurrences using a 2 comprehensive dataset collected from various urban junctions. Key traffic metrics such as vehicle flow, 3 average speed, speed differences, and occupancy were analyzed across different junction types to identify 4 high-risk areas. Advanced clustering techniques, including K-Means and DBSCAN, were employed to 5 detect patterns and hotspots of unsafe events. Local spatial analysis using Local Moran’s I and Geary’s C 6 highlighted significant clusters and spatial outliers, enhancing the spatial analysis framework. A Random 7 Forest Regressor was utilized to determine feature importance, identifying critical predictors of crash 8 occurrences, such as braking behavior, junction complexity, and monitoring duration. Multicollinearity 9 was assessed using Variance Inflation Factor (VIF) scores, ensuring the robustness of the models. 10 Principal Component Analysis (PCA) was also applied for dimensionality reduction, facilitating a more 11 straightforward interpretation of the data. Temporal trends were visualized to understand the variations in 12 traffic metrics over time. The results revealed significant variability in vehicle flow and speed across 13 different junctions, with high-risk areas identified based on speed fluctuations, occupancy rates, and 14 accident frequency. These insights provide a solid foundation for targeted safety interventions and policy-15 making aimed at improving road safety. The integration of these advanced analytical techniques with 16 detailed traffic data offers a comprehensive approach to understanding and mitigating unsafe driving 17 events and crashes.
ID | pc558 |
Presentation | |
Full Text | |
Tags |