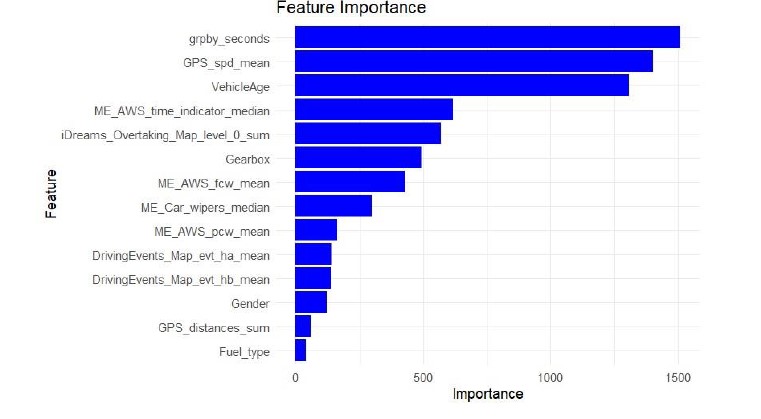
Task demand is the objective complexity of the task and arises out of a combination of features of 3 the environment, the behavior of other road users, control and performance characteristics of the vehicle. 4 On the other hand, coping capacity refers to the ability of drivers and road systems to manage and respond 5 effectively to various challenges and stressful situations encountered while driving. The aim of this study 6 was to identify crucial indicators of task complexity and coping capacity associated with crash risk through 7 machine learning techniques. Towards that end, data from an on-road driving experiment (involving 135 8 drivers) along with data from a simulator experiment (involving 55 drivers) were collected and analysed. 9 In order to fulfill these objectives, a feature importance algorithm extracted from Extreme Gradient 10 Boosting (XGBoost) was used to evaluate the significance of variables on forecasting STZ. Additionally, a 11 Neural Network model was implemented for real-time data prediction, taking into account the most 12 important and significant risk indicators. Furthermore, a comprehensive assessment of the performance of 13 three machine learning classifiers (i.e. Decision Trees, Random Forests and k-Nearest Neighbors) across 14 two distinct datasets (i.e. on-road and simulator experiment dataset) was performed to predict STZ levels 15 for headway. Results indicated that RF model outperformed the DT and kNN models across all metrics, 16 making it the most effective for predicting headway with accuracy up to 90%. It was also revealed that 17 Neural Networks demonstrated that the level of STZ can be predicted with an exceptional accuracy of up 18 to 89.8%.
ID | pc561 |
Presentation | |
Full Text | |
Tags |