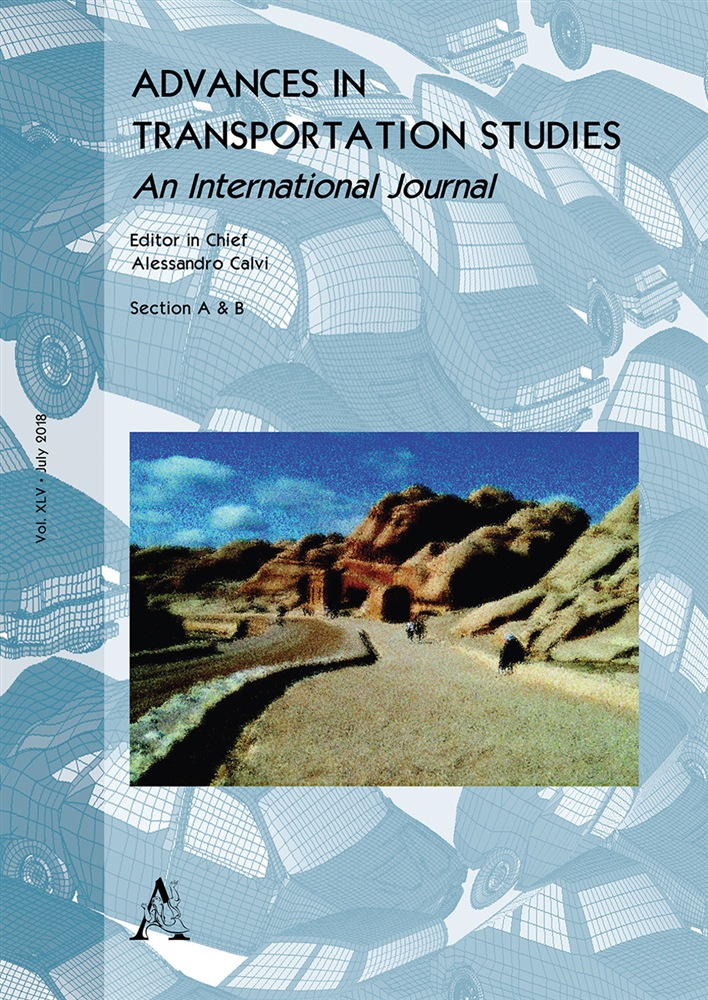
In road safety studies, spatial analyses are often implemented in order to reveal patterns of spatial variation and to examine the presence of spatial autocorrelation in road crash occurrences. Spatial analyses frequently employ exposure parameters in order to allow for comparisons between models. In this paper, meta-regression techniques are applied to three common exposure parameters (traffic volume/AADT, roadway length and vehicle distance traveled), to obtain quantitative estimates that several study characteristics impose on the values of their coefficients. Results indicate that the impact of traffic volume on crash counts was positively correlated with taking speed limit and road user age into consideration in spatial analyses, while the impact of road length on crash counts in spatial analyses was found to be higher in studies considering only fatal crashes. Finally, the impact of vehicle distance travelled on crash counts was found to be higher in county-level approaches as opposed to Traffic Analysis Zone-level approaches. The implication of findings is the quantification of coefficient discrepancies of exposure parameters between studies when including certain parameters as opposed to excluding them.
ID | pj171 |
Manuscript | |
Tags | machine learning, road infrastructure, statistical modelling |