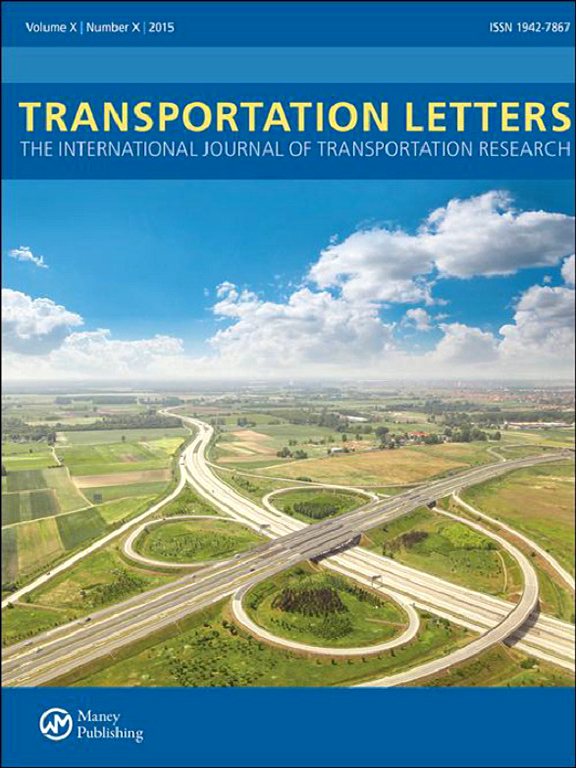
This study investigates factors influencing the severity of hit-and-run crashes using explainable machine learning techniques. A 5-year dataset from Victoria, Australia, was analyzed with CatBoost algorithms and SHAP values to highlight key severity factors. The presence of police at the crash scene emerges as the most critical determinant, underscoring the importance of law enforcement in mitigating severe crash outcomes. Crashes involving passenger vehicles and those on weekends were also linked to higher severity. The number of vehicles and total persons involved showed non-linear effects, with both low and high values associated with lower severity. Alcohol-related crashes and speed limit zones, while moderately important, revealed complex roles in severity prediction. These novel findings offer valuable insights for targeted interventions and policy-making to mitigate the impact of severe hit-and-run crashes and enhance road safety. In this way, policymakers can develop more effective strategies to reduce the impact of these phenomena.
ID | pj270 |
DOI | |
Tags |